Have you ever wondered if the rise of artificial intelligence (AI) in the field of epidemiology could potentially make the role of epidemiologists redundant? With AI’s ability to analyze vast amounts of data, it’s natural to question the future of this profession.
However, before jumping to conclusions, it is important to explore the intricacies of AI’s innovation in epidemiology and understand how it complements rather than replaces the work of epidemiologists.
Let’s delve into the impact of AI and whether or not it poses a threat to the job security of epidemiologists.
Key Takeaways
- AI revolutionizes epidemiology and enhances the capabilities of epidemiologists.
- While there is potential for job redundancy, AI cannot replace the expertise of epidemiologists.
- Continuous learning and adaptation are required for epidemiologists to keep up with rapid technological advancements.
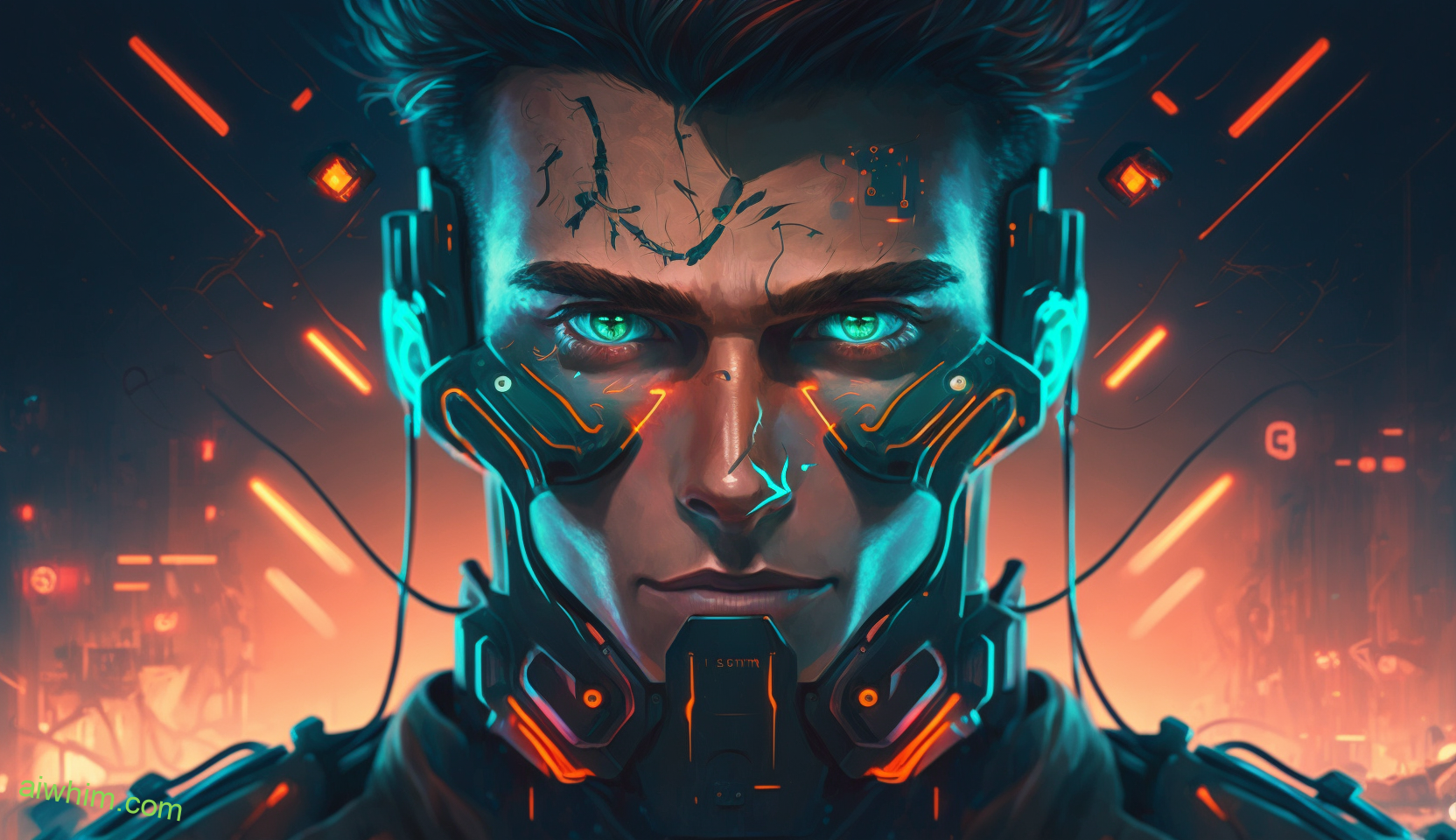
The Impact of AI on Epidemiology
AI is revolutionizing the field of epidemiology, making significant strides in reducing job redundancy for epidemiologists. The impact of AI on epidemiology can’t be overstated. With the advent of predictive modeling and AI-driven analysis, the way we understand and respond to diseases may be changing rapidly.
AI-driven interventions are also revolutionizing the way we respond to diseases. With AI, epidemiologists can develop personalized treatment plans for patients, considering factors such as genetic predisposition, lifestyle choices, and environmental exposures. This tailored approach may improve patient outcomes.
While AI is transforming the field of epidemiology, it’s important to recognize that it doesn’t replace the role of epidemiologists. Rather, it enhances their capabilities, allowing them to make data-driven decisions and focus their efforts on areas that require human expertise.
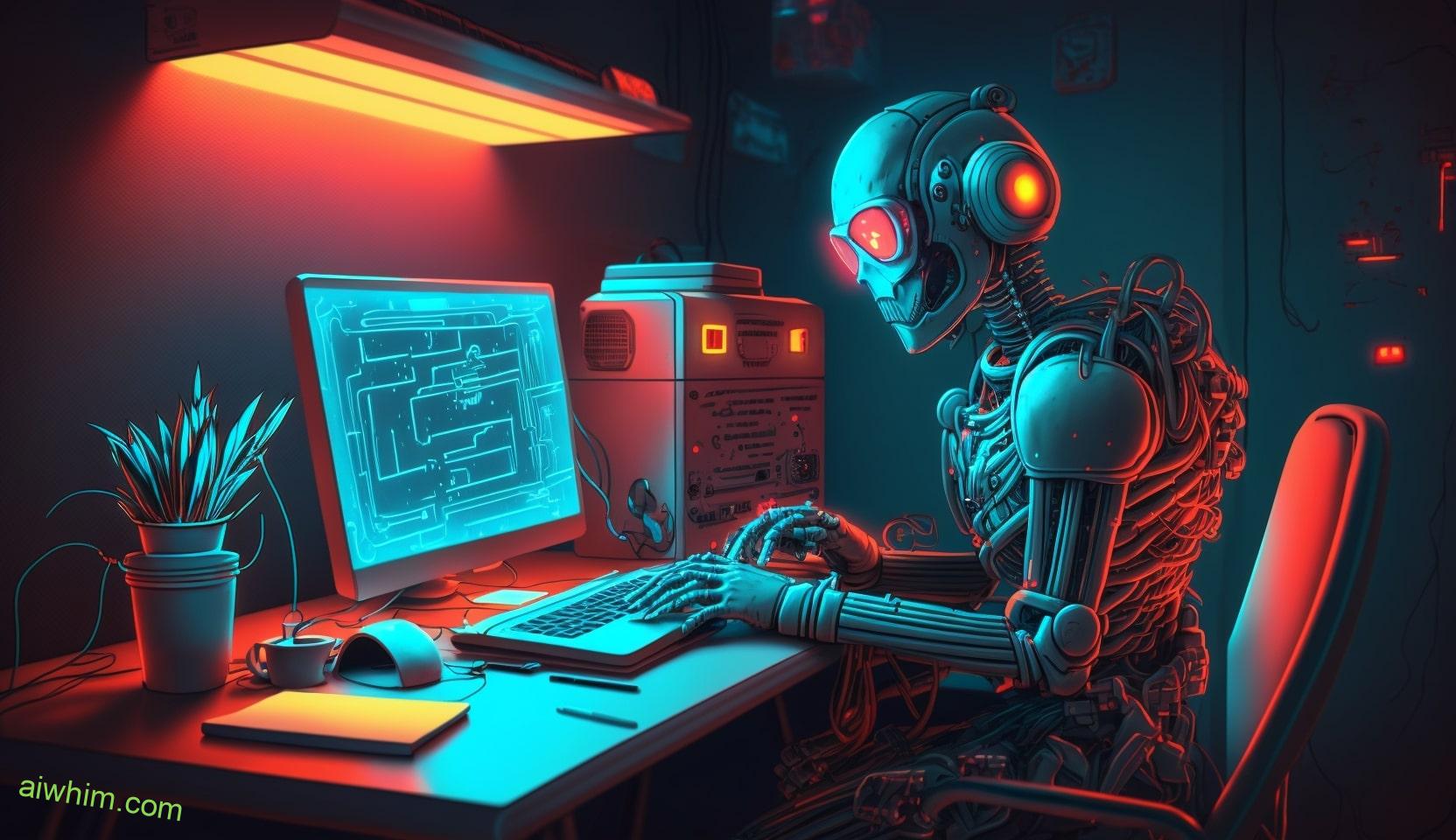
Challenges Faced by Epidemiologists in the Age of AI
Epidemiologists in the age of AI face a range of challenges that require adaptation and collaboration.
As artificial intelligence continues to advance, it’s natural to wonder about the impact it will have on job prospects in the field of epidemiology. While AI has the potential to revolutionize the way we collect and analyze data, it doesn’t necessarily mean that epidemiologists will become obsolete.
One of the challenges faced by epidemiologists is the need to keep up with the rapid pace of technological advancements. As AI becomes more sophisticated, epidemiologists must continuously update their skills to effectively utilize these new tools. This requires a willingness to learn and adapt to new technologies, as well as a commitment to staying informed about the latest developments in the field.
Another challenge is the potential for job redundancy. As AI becomes more capable of performing tasks traditionally done by epidemiologists, there’s a concern that some jobs may be automated. However, it’s important to remember that AI is a tool, not a replacement for human expertise. While AI can assist in data analysis and prediction, it can’t replace the critical thinking and decision-making skills of an experienced epidemiologist.
To overcome these challenges, collaboration is key. Epidemiologists must work closely with AI experts to develop and implement AI technologies that complement their work. By combining the strengths of both human expertise and AI capabilities, epidemiologists can enhance their ability to identify and respond to public health threats.
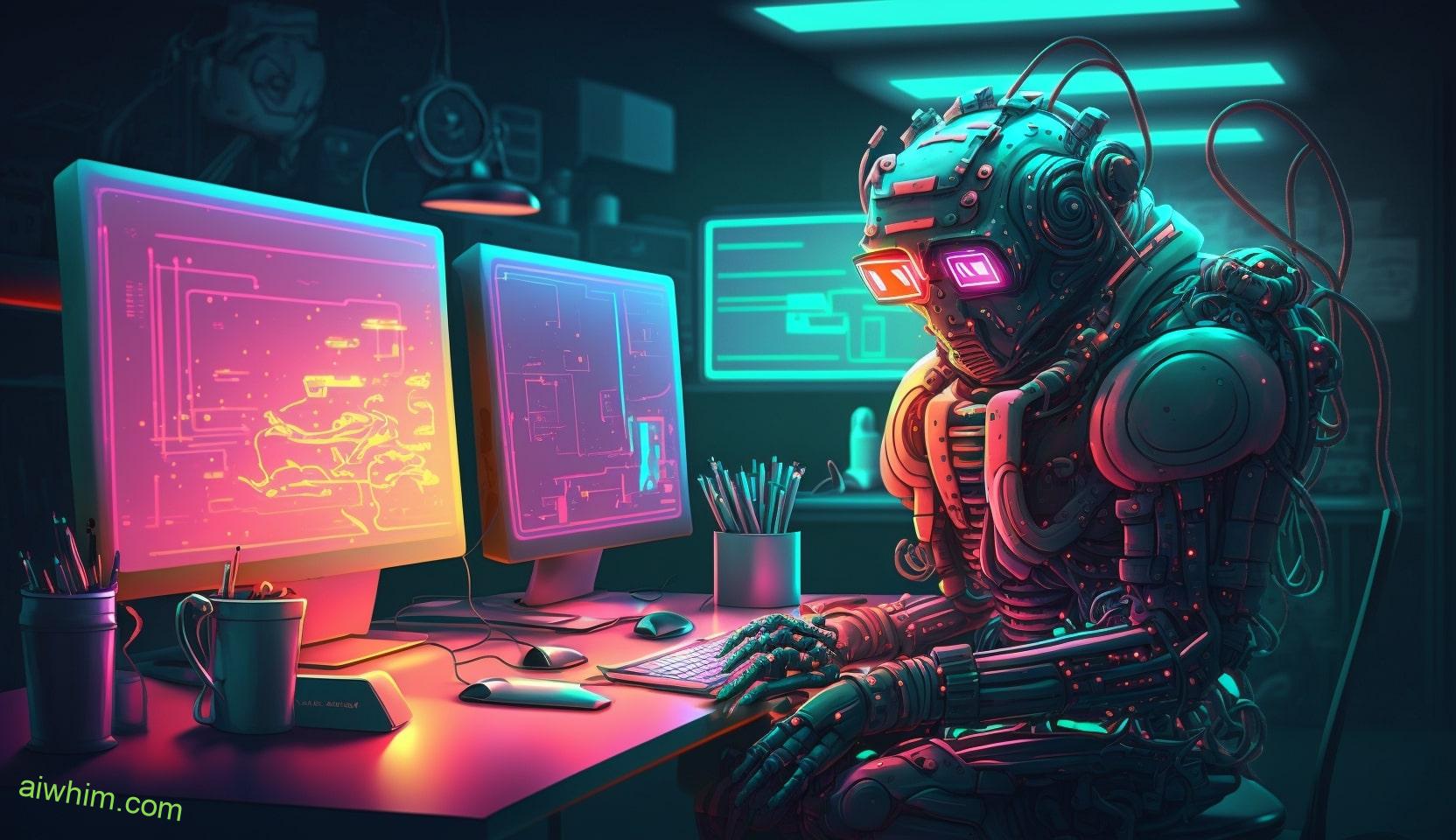
How AI Enhances Data Analysis in Epidemiology
As AI continues to advance, its impact on the field of epidemiology becomes evident through its ability to enhance data analysis. Artificial intelligence is revolutionizing public health by providing powerful tools for disease prevention. AI-powered technologies are transforming the way epidemiologists analyze data, leading to more accurate and efficient disease surveillance.
One of the key benefits of AI in epidemiology is its ability to process vast amounts of data quickly. Traditional methods of data analysis can be time-consuming and labor-intensive, but AI algorithms can analyze large datasets in a fraction of the time. This allows epidemiologists to identify patterns and trends in real-time, enabling faster response to disease outbreaks.
AI also improves the accuracy of data analysis by minimizing human error. Epidemiological data is often complex and requires careful interpretation. However, human analysts can inadvertently introduce errors or biases into their analysis. AI-powered tools, on the other hand, are designed to eliminate such errors, ensuring more reliable results.
Furthermore, AI can identify correlations and associations that may not be immediately apparent to human analysts. By analyzing vast amounts of data from various sources, AI algorithms can detect patterns and relationships that may have been overlooked by traditional methods. This can lead to new insights and discoveries in the field of epidemiology.
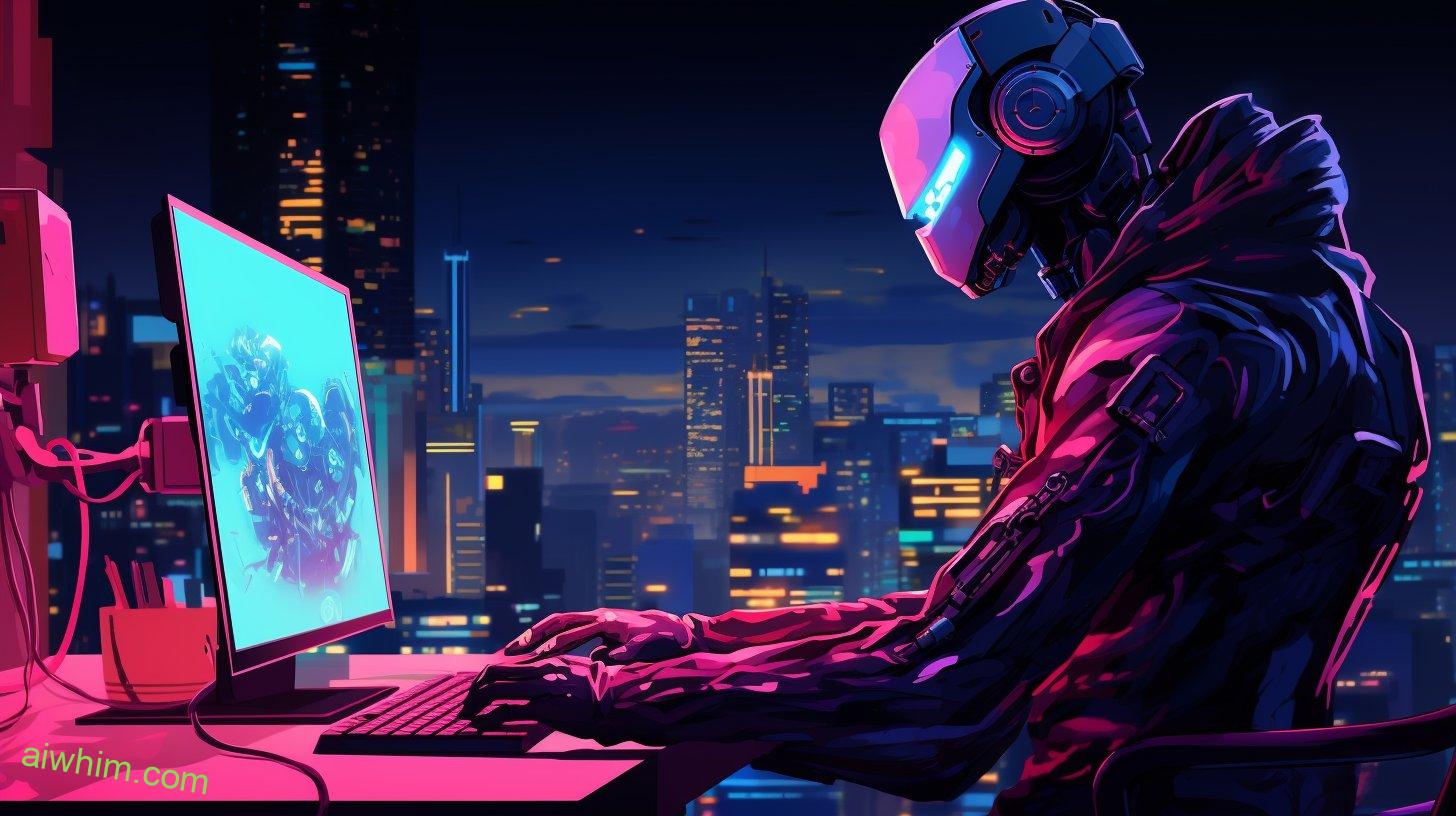
Ethical Considerations in AI-driven Epidemiology
Ethical considerations play a crucial role in the application of AI-driven epidemiology, ensuring responsible and fair use of these powerful technologies. As AI becomes increasingly integrated into the field of epidemiology, it’s important to be aware of the ethical implications that arise.
One such concern is data privacy. The use of AI in epidemiology often relies on large datasets containing sensitive information about individuals and populations. This raises concerns about how this data is collected, stored, and used. It’s essential to establish robust privacy measures to protect the confidentiality of individuals while still allowing for effective data analysis. This includes implementing strong data encryption, secure data storage systems, and strict access controls. Additionally, transparency in data collection and use is crucial to ensure that individuals are aware of how their data is being used and have the opportunity to provide informed consent.
Another ethical consideration in AI-driven epidemiology is the potential for bias in data analysis. AI algorithms are trained on large datasets, which may contain inherent biases that can influence the outcomes of the analysis. It’s important to address these biases and strive for fairness and equity in the results produced by AI-driven epidemiology. This can be achieved through careful selection and preprocessing of data, as well as ongoing monitoring and evaluation of the algorithms used.
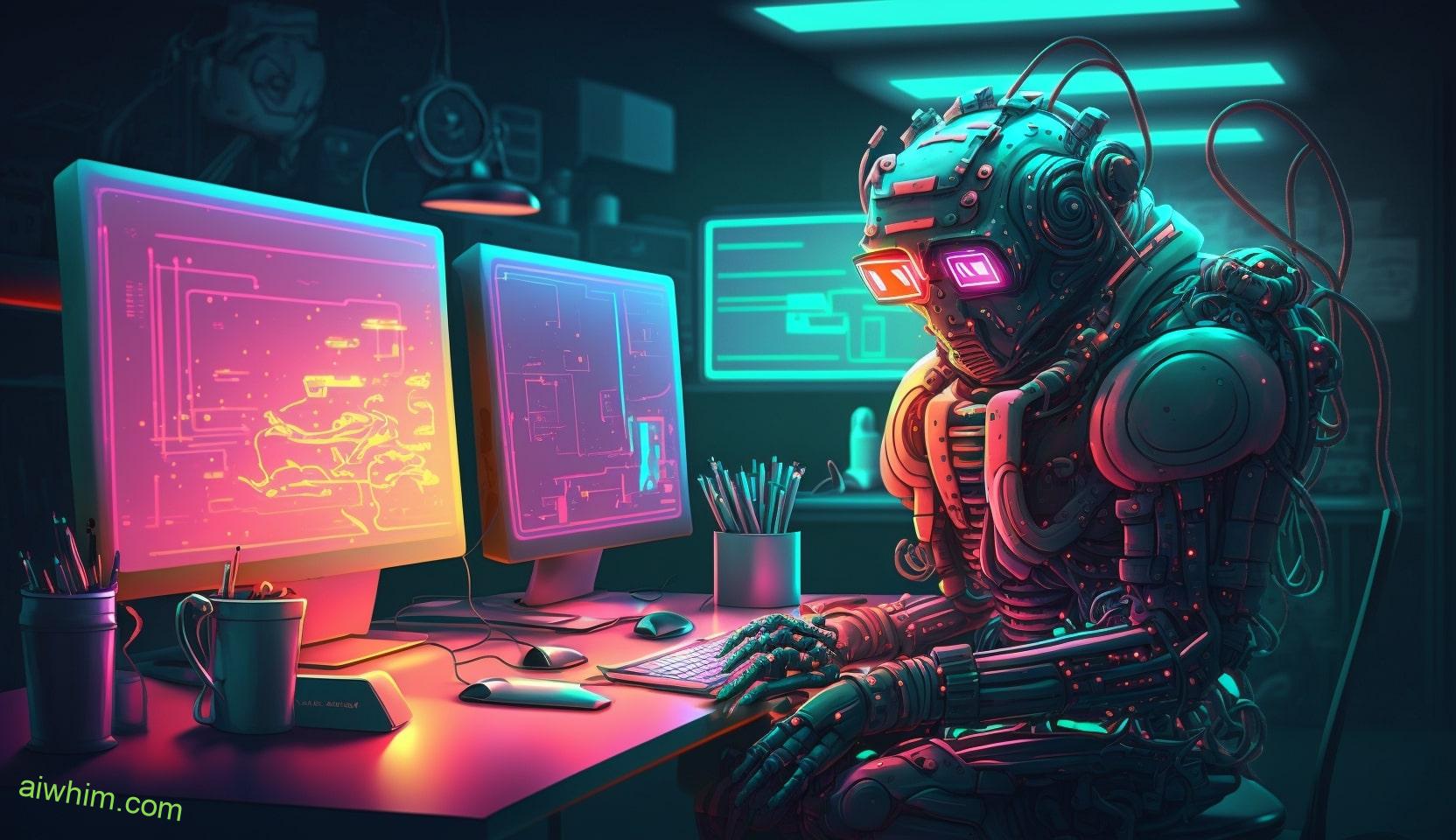
The Future of Epidemiology With AI
Incorporating AI into the field of epidemiology promises to revolutionize the way diseases are studied and managed. AI’s impact on public health is expected to be significant, with the potential to improve disease surveillance and enhance public health interventions. One of the key areas where AI can make a difference is in the automation of disease surveillance.
Currently, disease surveillance involves collecting and analyzing vast amounts of data from various sources, such as hospitals, laboratories, and public health agencies. This process can be time-consuming and resource-intensive. However, with the advent of AI, the task of disease surveillance can be automated, allowing for real-time monitoring and early detection of outbreaks.
AI algorithms can analyze data from multiple sources and identify patterns and trends that may indicate the presence of a disease outbreak. This can help public health agencies respond quickly and effectively, implementing measures to contain the spread of the disease and protect the population.
Furthermore, AI can assist in the prediction and modeling of disease outbreaks. By analyzing historical data, AI algorithms can identify risk factors and predict the likelihood of future outbreaks. This information can help public health officials allocate resources and develop targeted interventions to prevent the spread of diseases.
In addition to disease surveillance, AI can also play a role in managing and analyzing healthcare data. With the increasing availability of electronic health records, AI can help identify patterns and correlations within the data, providing valuable insights into disease prevalence, treatment efficacy, and patient outcomes.
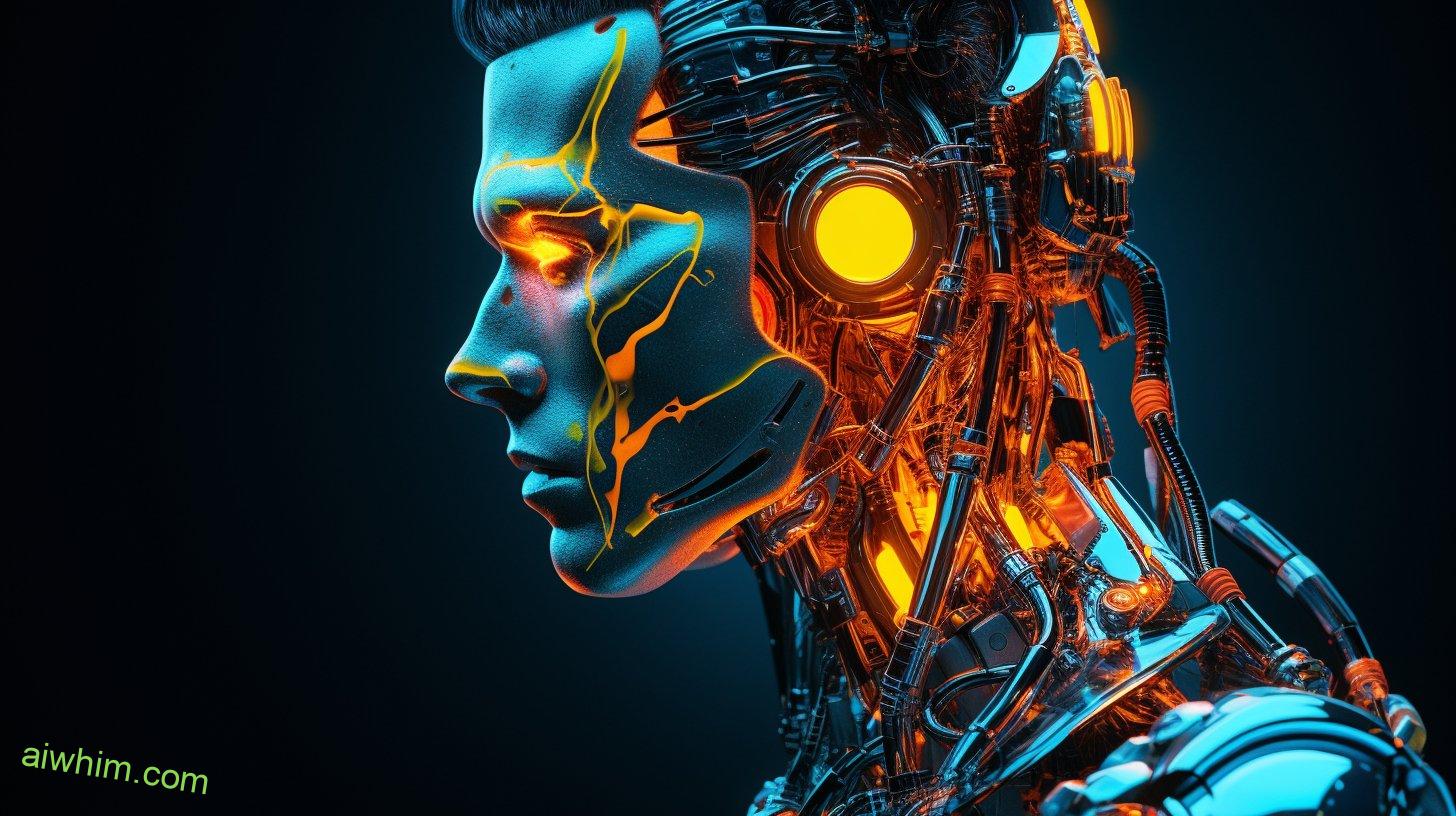
Collaborative Approach: Epidemiologists and AI Working Together
To foster a collaborative approach, epidemiologists and AI can combine their expertise to revolutionize disease surveillance and management. By working together, they can harness the power of collaborative research and data integration to address the challenges of modern healthcare effectively.
Here are four ways in which this collaboration can benefit both epidemiologists and AI:
- Improved data analysis: Epidemiologists possess domain knowledge and expertise in interpreting complex health data. By collaborating with AI, they can leverage its computational power to analyze vast amounts of data quickly and accurately. This combination allows for more comprehensive and insightful analysis, leading to better disease surveillance and management strategies.
- Enhanced predictive modeling: AI algorithms can process and analyze large datasets, enabling epidemiologists to create more accurate predictive models for disease outbreaks and trends. By integrating AI’s capabilities with their expertise, epidemiologists can make more informed decisions, allocate resources effectively, and implement targeted interventions to prevent and control the spread of diseases.
- Real-time monitoring: AI systems can continuously monitor various data sources, such as social media, online health forums, and electronic health records, for early signs of disease outbreaks. By collaborating with AI, epidemiologists can gain access to real-time data and insights, enabling them to respond promptly and efficiently to emerging threats.
- Automation of routine tasks: AI can automate time-consuming tasks, such as data cleaning and analysis, allowing epidemiologists to focus on more critical aspects of their work. This collaboration frees up their time and resources, enabling them to delve deeper into data interpretation, develop innovative research methodologies, and devise effective public health strategies.
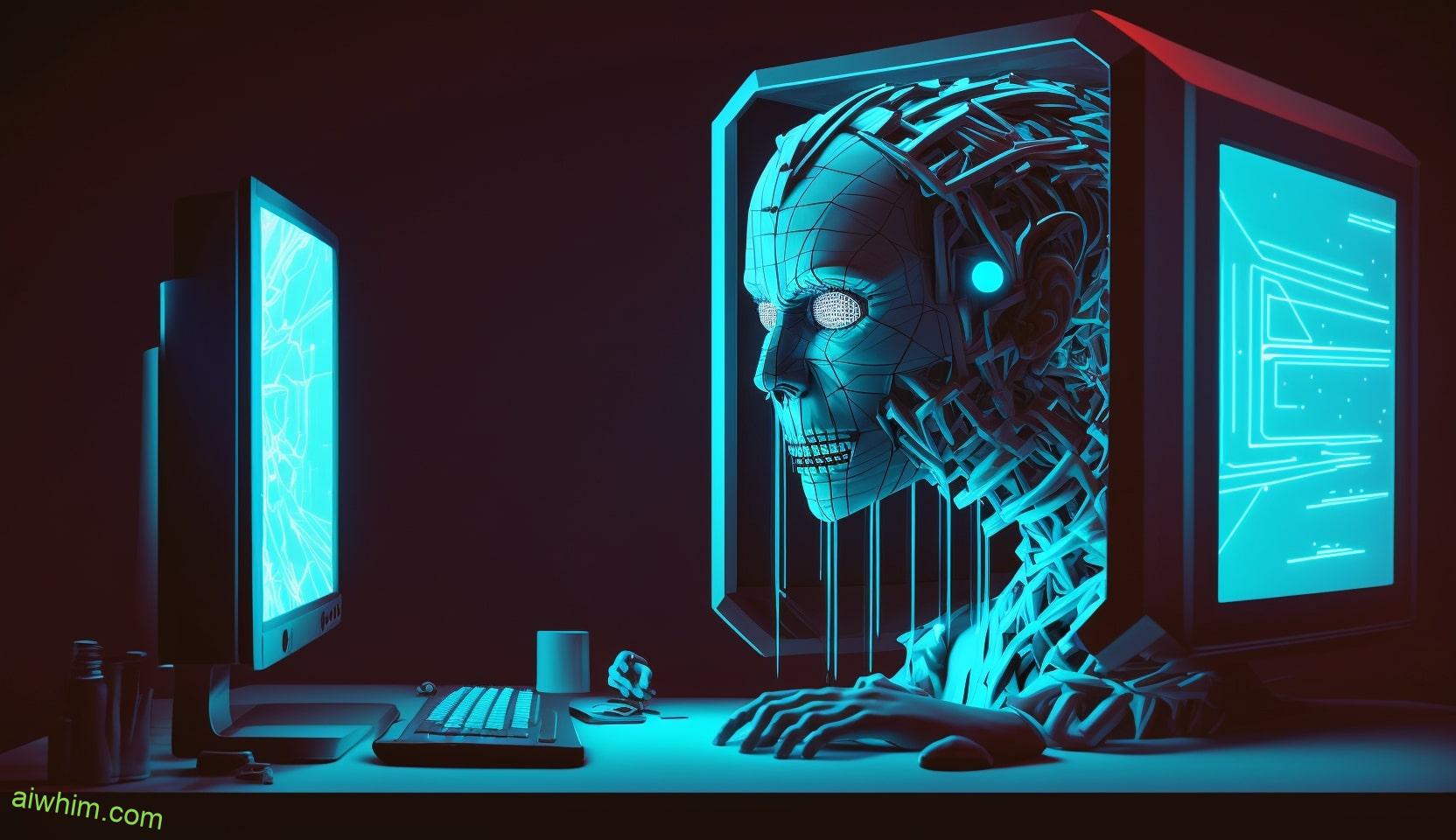
Training and Education for Epidemiologists in the AI Era
Epidemiologists must adapt their training and education to incorporate AI technologies in order to stay at the forefront of disease surveillance and management in the AI era. As technology continues to advance, it’s crucial for epidemiologists to embrace online learning platforms that offer courses and resources on AI-driven research.
Online learning provides the freedom and flexibility for epidemiologists to enhance their skills and knowledge without the constraints of traditional classroom settings. These platforms offer a wide range of courses, from introductory to advanced levels, that cover topics such as machine learning, data analysis, and AI algorithms. By taking advantage of these resources, epidemiologists can acquire the necessary expertise to effectively utilize AI technologies in their research and decision-making processes.
Incorporating AI-driven research into epidemiology training and education can greatly enhance disease surveillance and management. AI algorithms have the ability to analyze large datasets quickly and accurately, allowing epidemiologists to identify patterns and trends that may have otherwise been overlooked. This can lead to more efficient and targeted interventions, ultimately saving lives and improving public health outcomes.
Furthermore, integrating AI technologies into epidemiology training can also foster collaboration between human experts and machines. By understanding the capabilities and limitations of AI, epidemiologists can effectively leverage these technologies to augment their own expertise. This collaborative approach can lead to more robust and comprehensive research, as well as innovative solutions to complex public health challenges.
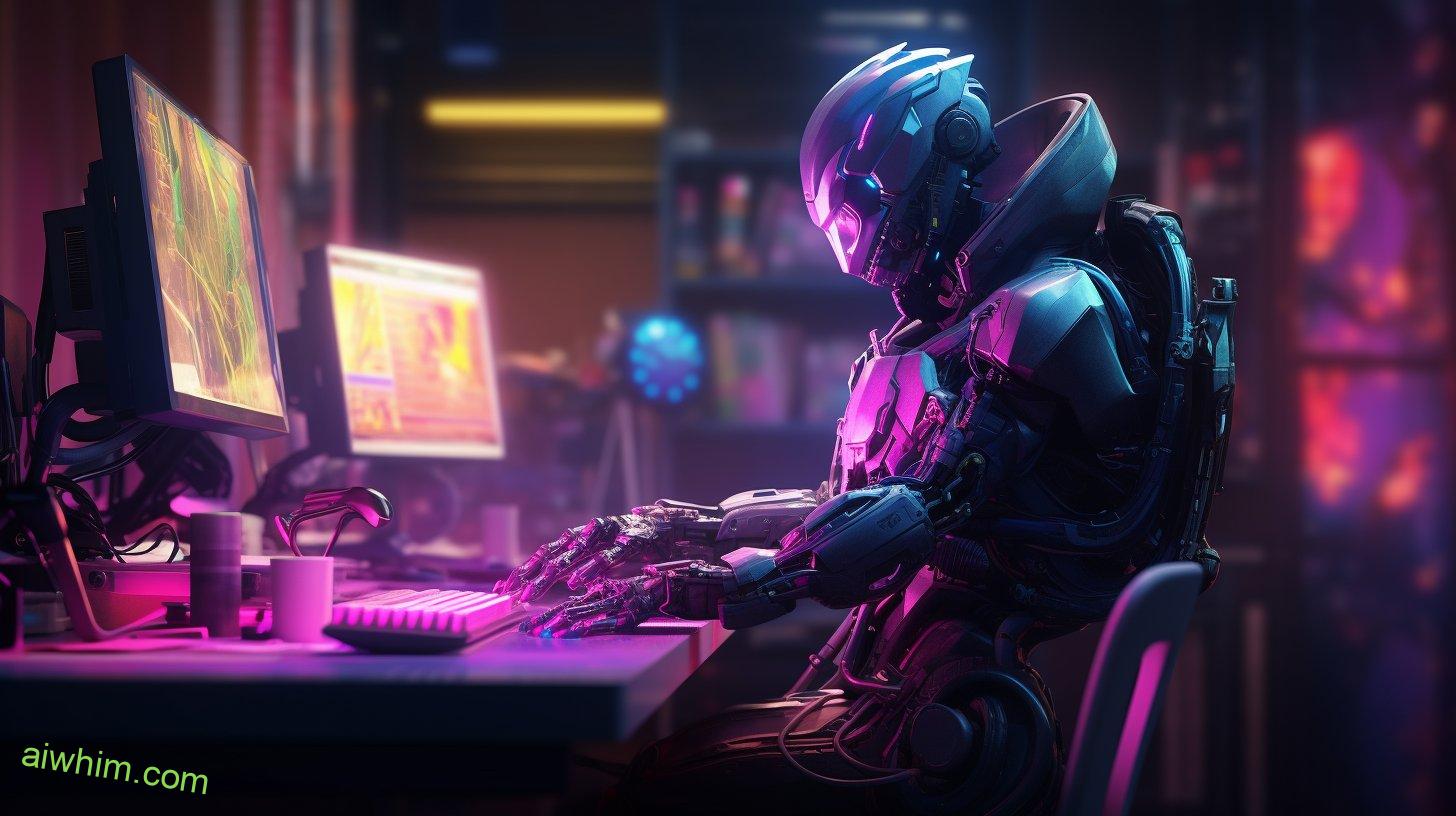
Limitations of AI in Epidemiology
As epidemiologists embrace AI-driven research and incorporate AI technologies into their training and education, it’s important to acknowledge the limitations of AI in the field of epidemiology. While AI has the potential to revolutionize the way we analyze and interpret data, there are certain limitations that need to be considered. Here are four key limitations of AI in epidemiology:
- Data Accuracy: One of the primary challenges with AI in epidemiology is ensuring the accuracy of the data being used. AI algorithms heavily rely on data inputs, and if the data is incomplete, biased, or of poor quality, it can lead to inaccurate results and conclusions. Therefore, it’s crucial to ensure the accuracy and reliability of the data before relying on AI for epidemiological analysis.
- Complexity of Disease Dynamics: Epidemiology is a complex field that involves studying the spread and impact of diseases on populations. AI algorithms may struggle to capture the intricate dynamics of disease transmission and progression accurately. The complexity of interactions between various factors, such as environmental conditions and individual behavior, poses a challenge for AI to fully grasp and model accurately.
- Ethical Considerations: AI algorithms are only as good as the data they’re trained on. There’s a risk that biased or discriminatory data could perpetuate biases in AI models, leading to unfair treatment or incorrect predictions. It’s essential to ensure that AI in epidemiology adheres to ethical guidelines and is used in a responsible and unbiased manner.
- Human Interpretation and Expertise: While AI can process and analyze vast amounts of data quickly, it lacks the human element of interpretation and expertise. Epidemiologists bring their domain knowledge, critical thinking, and experience to the table, which is essential for contextualizing and validating the results generated by AI. Ultimately, a combination of AI and human expertise is likely to be the most effective approach in epidemiological research and decision-making.
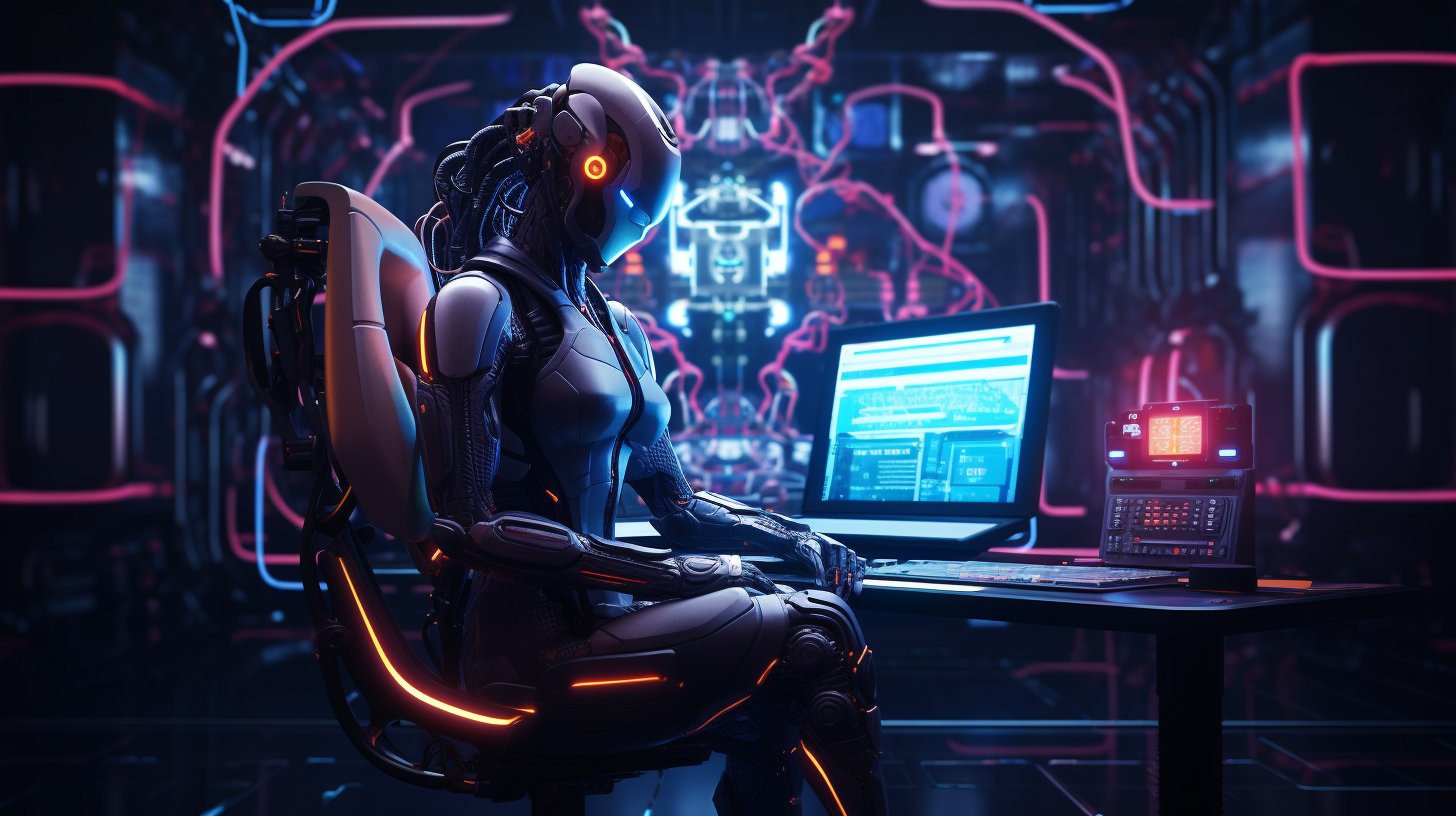
In Sum: The Coexistence of Epidemiologists and AI
The coexistence of AI and epidemiologists is essential for advancing the field of epidemiology. While AI has shown great potential in analyzing big data and identifying patterns, it can’t replace the expertise and intuition of human epidemiologists. The collaborative approach between AI and epidemiologists can lead to more accurate and comprehensive insights into disease patterns, risk factors, and preventive measures.
Epidemiologists play a crucial role in designing and conducting studies, collecting and analyzing data, and interpreting the results. Their deep understanding of the complex interplay between biological, environmental, and social factors is invaluable in uncovering the underlying causes of diseases. AI, on the other hand, can process vast amounts of data quickly and identify patterns that may go unnoticed by human researchers. By combining the strengths of both, a more holistic understanding of diseases can be achieved.
The coexistence of AI and epidemiologists also allows for a more efficient allocation of resources. AI can assist in prioritizing research areas, identifying high-risk populations, and optimizing interventions. This collaboration can lead to more targeted and effective public health strategies, ultimately saving lives and reducing healthcare costs.
Moreover, the collaborative approach between AI and epidemiologists promotes transparency and accountability. By involving human experts in the decision-making process, the potential biases and limitations of AI algorithms can be identified and addressed. This ensures that the findings and recommendations generated by AI are reliable and trustworthy.
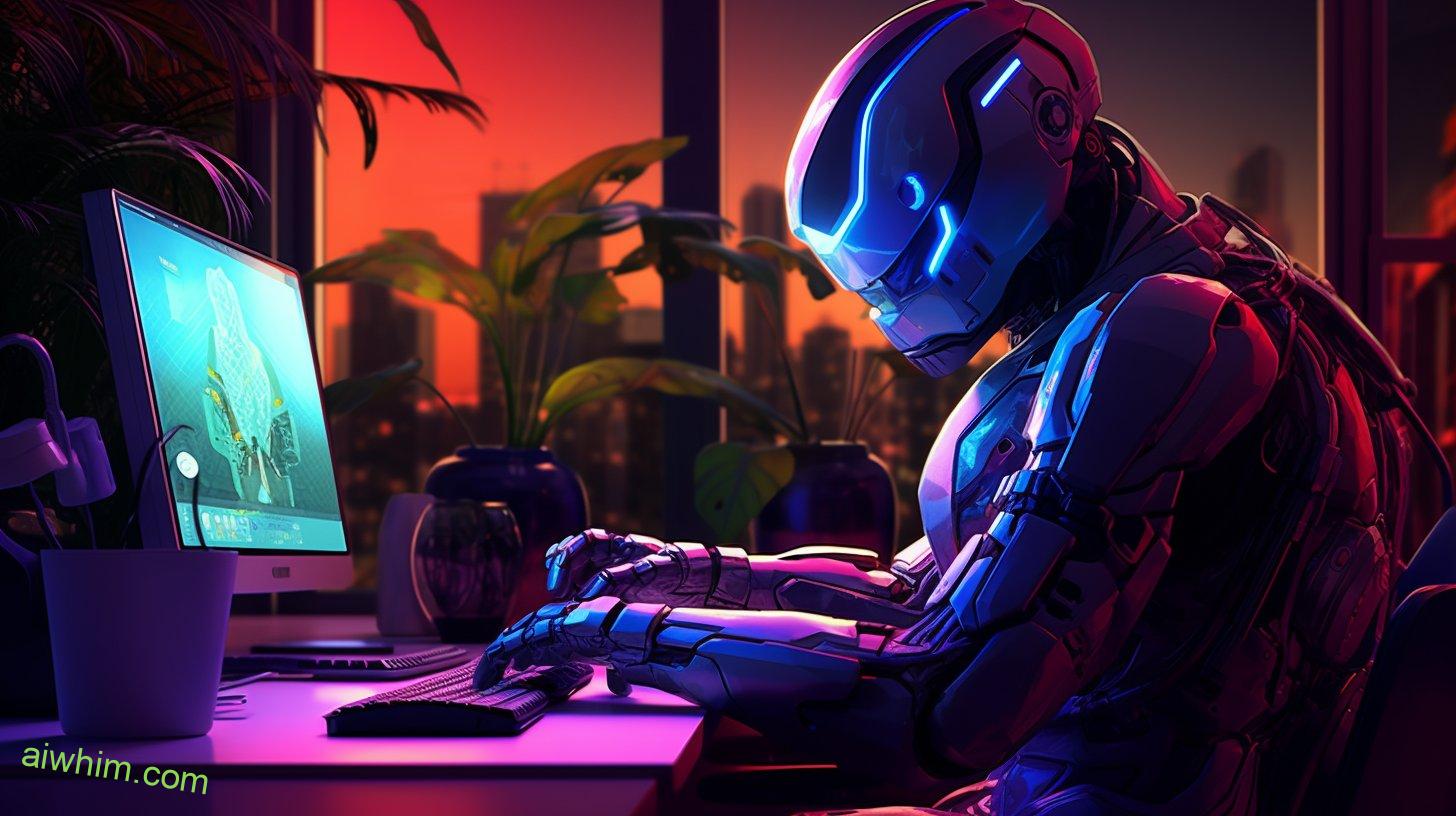
Frequently Asked Questions
How Does AI Impact the Job Market for Epidemiologists?
AI’s role in the job market and its impact on epidemiologists is significant. It has the potential to automate certain tasks, leading to job redundancy. However, it also opens up new opportunities for collaboration and innovation in the field.
How Does AI Contribute to Predicting Disease Outbreaks?
Predictive models and machine learning algorithms in AI contribute significantly to predicting disease outbreaks. These advanced technologies analyze vast amounts of data quickly and accurately, providing valuable insights that can help prevent and control the spread of diseases.
What Challenges Do Epidemiologists Face in the Age of Ai?
In the age of AI, epidemiologists face challenges in navigating ethical considerations and adapting to the future of their field. But fear not, for with freedom comes the opportunity for growth and innovation.
How Does AI Enhance Data Analysis in Epidemiology?
AI enhances data analysis in epidemiology by streamlining processes and identifying patterns in vast datasets. It plays a crucial role in disease surveillance, improving accuracy and efficiency. Embrace the freedom AI offers to revolutionize epidemiological research.
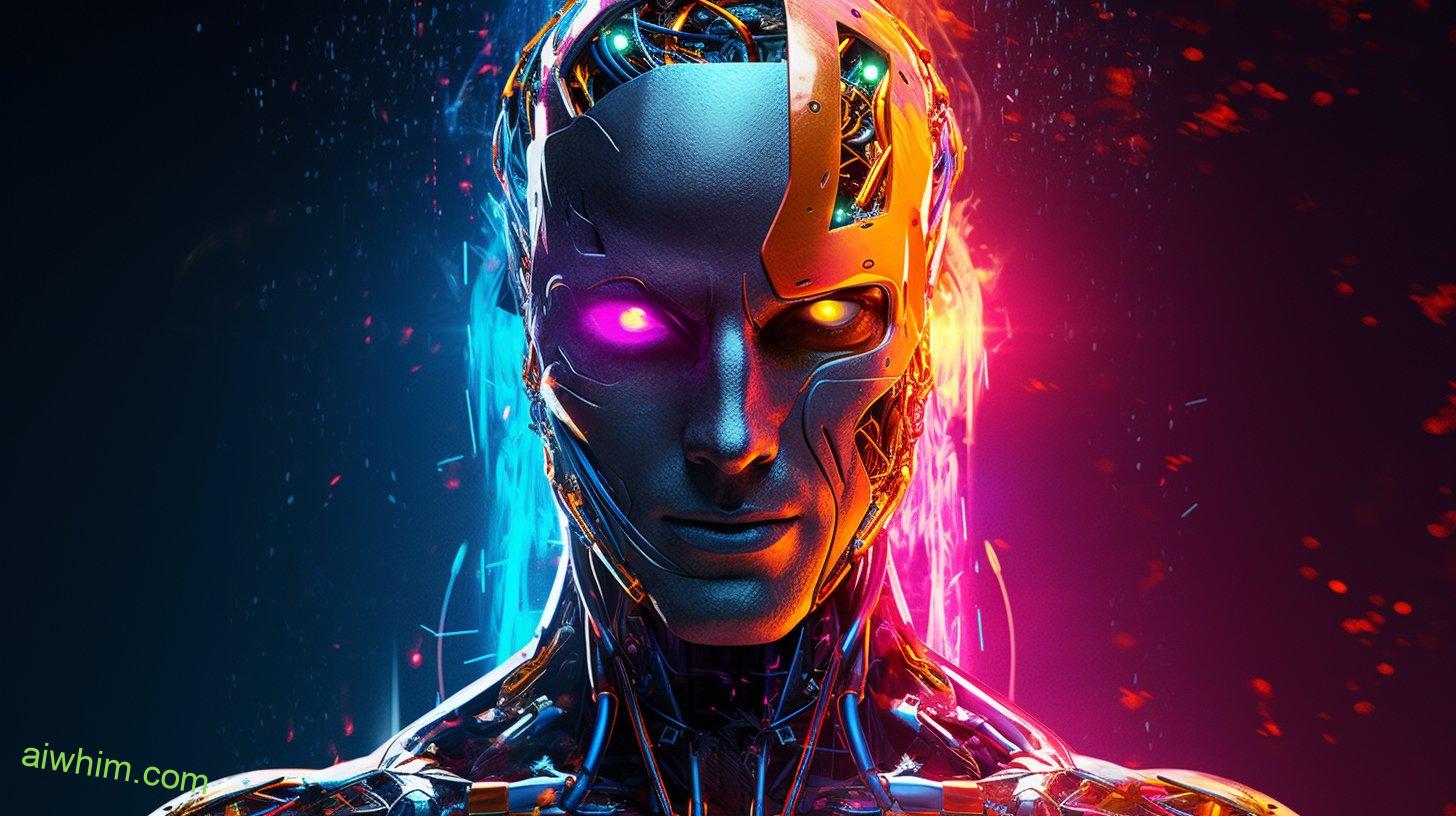
Conclusion
In conclusion, while AI has the potential to enhance data analysis in epidemiology, it’s unlikely to completely replace human epidemiologists.